7. Schema Loader Module – Load Embedded or External Schema¶
A Schema Loader loads the attributes of a schema from a source document. There are a variety of sources.
- The first row of a sheet within a workbook. This version has to be injected into workbook processing so that the first row is separated from the data rows.
- A separate sheet of a workbook. This version requires a sheet name.
- A separate workbook. This, too, requires a named sheet.
- COBOL Code. We’ll set this aside as a subclass so complex it requires it’s own module.
A schema loader is paired with a specific kind of sheet.Sheet
.
A workbook requires a schema, which requires a schema loader. A schema loader depends on a meta-workbook. Ideally that meta-workbook has an emedded schema, but it may have an external schema, meaning we could have a meta-schema required load the schema for the application data. Sheesh.
First, let’s hope that doesn’t happen. Second, the circularity is resolved by making it the responsibility of the the application to handle schema loading.
7.1. Embedded Schema Use Case¶
A sheet.EmbeddedSchemaSheet
requires a loader class.
The loader will
- Be built with the sheet as an argument.
- Be interrogated for the schema.
- Be interrogated for the rows.
The most typical case is the single-header-row case.
In some cases, the loader is actually a a rather sophisticated parser that paritions the data into the embedded schema and the data rows.
with Workbook( name ) as wb: sheet = self.wb.sheet( 'Sheet2', stingray.sheet.EmbeddedSchemaSheet, loader_class= stingray.schema.loader.HeadingRowSchemaLoader ) for row in sheet.rows(): process the row
7.2. External Schema Use Case¶
A sheet.ExternalSchemaSheet
requires a schema.
In the typical case, the external schema file has an emedded meta-schema.
The first row has appropriate column names.
This requires a subclass of schema.loader.ExternalSchemaLoader
to properly map the names that were found onto the attributes of the schema.Attribute
class.
When the embedded meta-schema has unusual names, then a builder must be defined
to map the names that are found in the schema and build an schema.Attribute
instance.
with open_workbook( schema_name ) as schema_wb:
esl= stingray.schema.loader.ExternalSchemaLoader( schema_wb, "Schema" )
schema= esl.schema()
with Workbook( name, schema=schema ) as wb:
sheet = self.wb.sheet( 'Sheet2',
stingray.sheet.ExternalSchemaSheet,
schema= schema )
counts= process_sheet( sheet )
pprint.pprint( counts )
7.3. Manual Schema Use Case¶
Also, a manually-defined schema.Schema
can be built rather than being loaded.
schema= stingray.schema.Schema(
stingray.schema.Attribute( name='Column #1' ),
stingray.schema.Attribute( name='Key' ),
stingray.schema.Attribute( name='Value' ),
stingray.schema.Attribute( name='Etc.' ),
)
7.4. Model¶
http://yuml.me/diagram/scruffy;/class/
#schema-loader,
[Schema]<>-[Attribute],
[SchemaLoader]-builds->[Schema],
[SchemaLoader]^[HeadingRowSchemaLoader],
[SchemaLoader]^[ExternalSchemaLoader],
[ExternalSchemaLoader]-reads->[Workbook],
[HeadingRowSchemaLoader]-reads->[Sheet].
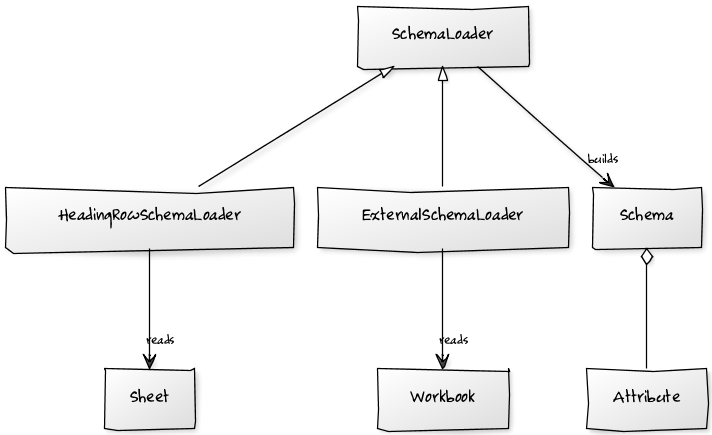
7.5. Overheads¶
We depend on schema
, cell
and sheet
.
"""stingray.schema.loader -- Loads a Schema from a row of a Sheet or
from a separate Sheet. This is extended to load COBOL schema
from DDE files.
"""
from stingray.schema import Schema, Attribute
import stingray.cell
import stingray.sheet
import warnings
7.6. No Schema Exception¶
In some circumstances, we can’t load a schema. The most common situation
is a HeadingRowSchemaLoader
which is applied to an empty workbook sheet.
No rows means no schema.
class NoSchemaFound( Exception ):
pass
The default behavior is to simply write a warning for an empty sheet. The lack of a schema means there’s no data, also, and 99% of the time, silently ignoring an empty sheet is desirable.
7.7. Schema Loader¶
-
class
schema.loader.
SchemaLoader
¶ A Schema Loader has one mandatory contract: It must load the schema.
A subclass may add a second contract, For example, an embedded schema loader will also return the non-schema rows.
-
sheet
¶ The
Sheet
associated with this schema.
-
row_iter
¶ An iterator over the rows of this sheet; used to pick rows that belong to the header, separate from the rows that belong to data.
-
class SchemaLoader:
"""Locate schema information. Subclasses handle
all of the variations on schema representation.
"""
def __init__( self, sheet ):
"""A simple :py:class:`Sheet` instance."""
self.sheet= sheet
self.row_iter= iter( self.sheet.rows() )
def schema( self ):
"""Scan the sheet to get the schema.
:return: a :py:class:`Schema` object."""
return NotImplemented
def rows( self ):
"""Iterate all (or remaining) rows."""
return self.row_iter
7.8. Embedded Schema Loader¶
-
class
schema.loader.
HeadingRowSchemaLoader
¶ In many cases, the schema is first-row column titles or something similar. As we noted above,
csv.DictReader
supports this simple case.All other cases have to be handled with something a bit more sophisticated. The
schema.loader.SchemaLoader
can be further subclassed to provide for more complex schema definitions buried in the rows of a sheet.This means that we must make the schema parsing an application-provided plug-in that the Workbook uses when instantiating each Sheet.
class HeadingRowSchemaLoader( SchemaLoader ):
"""Read just the first row of a sheet to get embedded
schema information."""
def schema( self ):
"""Try to get the schema from row one. Remaining rows are data.
If the sheet is empty, emit a warning and return ``None``.
"""
try:
row_1= next( self.row_iter )
attributes = (
dict(name=c.to_str()) for c in row_1
)
schema = Schema(
*(Attribute(**col) for col in attributes)
)
return schema
except StopIteration:
warnings.warn( "Empty sheet: no schema present" )
We’ll open a sheet.Sheet
with a specific loader.
sheet= stingray.sheet.EmbeddedSchemaSheet(
self.wb, 'The_Name',
loader_class=HeadingRowSchemaLoader )
-
class
schema.loader.
NonBlankHeadingRowSchemaLoader
¶ In many cases, we’d like to suppress the empty rows that are an inevitable feature of workbook sheets.
Note that this doesn’t work well for COBOL or Fixed format files, since an “empty” row may be difficult to discern.
class NonBlankHeadingRowSchemaLoader( HeadingRowSchemaLoader ):
def __init__( self, sheet ):
"""A simple :py:class:`Sheet` instance."""
self.sheet= sheet
self.row_iter= self.non_blank( self.sheet.rows() )
def non_blank( self, rows ):
for r in rows:
if all( c.is_empty() for c in r ):
continue
yield r
7.9. External Schema Loader¶
-
class
schema.loader.
ExternalSchemaLoader
¶ In some cases, the data workbook is described by a separate schema workbook, or a separate sheet within the data workbook. In these cases, the other sheet (or file) must be parsed to locate schema information.
In the case of a fixed format file, we must examine a separate file to load schema information. This additional schems file may be in COBOL notation, leading to a more complex parser. See COBOL Loader Module – Parse COBOL Source to Load a Schema.
The layout of the schema, of course, will be highly variable, so the “meta-schema” must be adjusted to the actual file.
Note, also, that the schema loader is – itself – a typical of schema-based reader. It has a number of common features.
- A dictionary-based “builder”,
schema.loader.ExternalSchemaLoader.build_attr()
, to handle Logical Layout. This transforms the input “raw” dictionary ofcell.Cell
instances to an application dictionary of proper Python objects. See The Stingray Developer’s Guide. - An iterator,
schema.loader.ExternalSchemaLoader.attr_dict_iter()
, that provides “raw” dictionaries from each row (based on the schema) to the builder to create application dictionaries. - The overall function,
schema.loader.ExternalSchemaLoader.schema()
, that iterates over application objects built from application dictionaries.
-
workbook
¶ The overall Workbook that we’re parsing to locate schema information.
-
Sheet
¶ A specific sheet within that workbook.
- A dictionary-based “builder”,
class ExternalSchemaLoader( SchemaLoader ):
"""Open a workbook file in a well-known format.
Build a schema with attribute name, offset, size and type
information. The type is a string that names the
type of cell to create.
The meta-schema must be embedded as the first line of the schema sheet.
The assumed meta-schema is the following::
Schema(
Attribute("name",create="TextCell"),
Attribute("offset",create="NumberCell"),
Attribute("size",create="NumberCell"),
Attribute("type",create="TextCell"),
)
If the meta-schema has different names, then a subclass with
a different :py:meth:`build_attr` is required to map the actual
source columns to the attributes of a :py:class:`Attribute`.
Offsets are typically 1-based.
"""
def __init__( self, workbook, sheet_name='Sheet1' ):
self.workbook, self.sheet_name = workbook, sheet_name
self.sheet= self.workbook.sheet( self.sheet_name, stingray.sheet.EmbeddedSchemaSheet,
loader_class= HeadingRowSchemaLoader )
-
ExternalSchemaLoader.
build_attr
(row)¶ There’s potential for a great deal of variability in schema definition. Consequently, this
build_attr
method is merely a sample that covers one common case.
base= 1
type_to_cell = {
'text': "TextCell",
'number': "NumberCell",
'date': "DateCell",
'boolean': "BooleanCell",
}
@staticmethod
def build_attr( row ):
"""Build application dictionary from raw dictionary.
"""
try:
offset= row['offset'].to_int()-ExternalSchemaLoader.base
except KeyError:
offset= None
try:
size= row['size'].to_int()
except KeyError:
size= None
try:
type_name= row['type'].to_str()
create= ExternalSchemaLoader.type_to_cell[type_name]
except KeyError:
create= stingray.cell.TextCell
return dict(
name= row['name'].to_str(),
offset= offset,
size= size,
create= create,
)
Schema loading involves a process of
- Iterating through the source rows as dictionaries.
- Build each raw row as a source dictionary.
- Build an standardized attr dictionary from the source dictionary.
This mapping, implemented by
schema.loader.ExternalSchemaLoader.build_attr()
is subject to a great deal of change without notice.
- Building each
schema.Attribute
from the dictionary.
-
ExternalSchemaLoader.
attr_dict_iter
(sheet)¶ Iterate over application dicts based on raw dicts built by the schema of the sheet.
def attr_dict_iter( self, sheet ):
"""Iterate over application dicts based on raw dicts
built by the schema of the sheet."""
return (
ExternalSchemaLoader.build_attr(r)
for r in sheet.schema.rows_as_dict_iter(sheet)
)
-
ExternalSchemaLoader.
schema
()¶ Scan a file to get the schema.
Returns: a Schema
object
def schema( self ):
"""Scan a file to get the schema.
:return: a :py:class:`Schema` object."""
self.row_iter= iter( [] )
source_dict = self.attr_dict_iter( self.sheet )
schema= Schema(
*(Attribute(**row) for row in source_dict)
)
return schema
7.10. Worst-Case Loader¶
-
class
schema.loader.
BareExternalSchemaLoader
¶ This is a degenerate case loader where the schema sheet (or file) doesn’t have an embedded schema on line one of the sheet.
class BareExternalSchemaLoader( SchemaLoader ):
"""Open a workbook file in a well-known format. Apply a schema parser
to the given sheet (or file) to build a schema.
The meta-schema is hard-coded in this class because the given
sheet has no headers.
"""
schema= Schema(
Attribute("name",create="TextCell"),
Attribute("offset",create="NumberCell"),
Attribute("size",create="NumberCell"),
Attribute("type",create="TextCell"),
)
def __init__( self, workbook, sheet_name='Sheet1' ):
self.workbook, self.sheet_name = workbook, sheet_name
self.sheet= self.workbook.sheet( self.sheet_name, stingray.sheet.ExternalSchemaSheet,
schema= self.schema )
7.11. Parsing and Loading a COBOL Schema¶
One logical extension to this is to parse COBOL DDE’s to create a schema that allows us to process a COBOL file (in EBCDIC) directly as if it were a simple workbook.
We’ll delegate that to COBOL Loader Module – Parse COBOL Source to Load a Schema, since it’s considerably more complex than simply loading rows from a sheet of a workbook.